- Blog
- The Future of Design: Exploring Generative AI Applications in Architecture and Urban Planning
- AI Articles , Articles ,
- April 18, 2023
Architecture and urban planning are essential components of our built environment, shaping the spaces in which we live, work, and play. These fields have a rich history, with roots in ancient civilizations and evolving through various design styles and approaches. As our societies grow and urbanization continues, the need for sustainable and innovative solutions in architecture and urban planning becomes more critical than ever.
However, despite advancements in technology and design tools, these industries still face many challenges, such as optimizing limited space, ensuring energy efficiency, reducing environmental impact, and adhering to ever-changing regulations. AI can shape the future of designs and architecture and to address the above challenges and create a more sustainable future, architects and urban planners are increasingly turning to generative AI. This powerful technology has the potential to revolutionize the way we design and build our cities, offering new solutions to the industry’s most pressing problems.
Generative AI for Architecture
Generative AI is an emerging technology that has the potential to revolutionise the way architects and engineers design and model complex structures. By using algorithms to create designs and models that meet specific requirements, Generative AI enables architects and engineers to generate multiple design options, explore design solutions that might have been difficult to visualise or conceive otherwise and optimise their designs for various factors such as energy efficiency, sustainability, and safety.
Here are some real use cases of Generative AI in architecture:
- Designing Energy-efficient Buildings: By developing models that optimise variables like building orientation, glazing ratios, shading, and insulation, generative AI can aid architects and engineers in designing energy-efficient structures. Building orientation, or the direction a building faces, can have an impact on things like ventilation, solar heat gain, daylighting, and other aspects. Glazing ratios, or how much glass is on a building’s front, can have an effect on things like energy efficiency and thermal comfort. The term “shading” describes elements like awnings or louvres that can lower solar heat gain and increase energy efficiency. Insulation is the term for the substance used to stop heat from transferring from a building’s interior to its exterior.
- Enhancing Accessibility and Safety: Enhancing accessibility and safety in urban settings is another application for generative AI. While safety relates to elements like the security of bicyclists and pedestrians, the availability of emergency services, and the reduction of crime, accessibility refers to the ease with which people may move about and navigate an area. By maximising aspects like connection, pedestrian and bicycle pathways, and lighting, generative AI algorithms may produce designs for streets and public places that prioritise accessibility and safety.
- Sustainability and Environmental Factors: Generative AI can help architects and engineers design sustainable buildings by optimising various environmental factors such as daylighting, ventilation, and thermal comfort.
Optimization of Building Structure:
Additionally, generative AI can also assist architects and engineers in optimising building structures. When a building is structurally efficient, it can withstand outside pressures like wind and earthquakes while utilising the least amount of materials possible. Algorithms that use generative AI can produce building structure designs that are optimised for things like structural effectiveness, material waste, and aesthetic appeal.
Applications of Generative AI in Urban Planning
Urban planning involves designing and managing the physical and social aspects of cities, towns, and other urban areas. This includes everything from transportation systems to public spaces to housing and commercial developments. Generative AI can play a crucial role in urban planning by helping planners and designers create more efficient, sustainable, and livable urban environments. Here are some real-world examples of how Generative AI is being used in urban planning:
- Optimising Traffic Flow: One way that generative AI can be applied in urban planning is by optimizing traffic flow. This entails enhancing the flow of traffic in metropolitan settings, such as by lowering congestion and delays. By analysing real-time traffic data and predicting traffic patterns, generative AI can aid in this process. Adaptive traffic signal timing and dynamic lane assignments are two essential traffic management tactics that can be improved with the help of this data. Adaptive traffic signal timing involves using sensors and real-time traffic data to adjust the timing of traffic signals in real-time. This can help to reduce congestion and improve traffic flow. Dynamic lane assignments involve temporarily changing the direction of traffic lanes during times of high traffic to improve traffic flow.
- Enhancing Public Space Design: Generative AI can be used to enhance public space design by generating designs that prioritise vital factors such as accessibility, safety, and user preferences. Accessibility is a critical factor when it comes to public space design, as it ensures that people with disabilities can access and enjoy public spaces. Safety is yet another important factor, as public spaces that are well-lit and incorporate features such as seating and surveillance cameras are less likely to be targeted by crime. User preferences are also important, as public spaces that are designed to meet the needs and preferences of users are more likely to be well-used and well-maintained.
- Streamlining Building Permitting: Building permitting is a complex process that involves obtaining approvals from various city officials and departments. Generative AI can be used to streamline the permitting process by generating digital 3D models of proposed buildings that can be quickly reviewed by city officials. This can reduce the time and resources required to obtain building permits, speeding up the development process and reducing costs.
- Improving Disaster Preparedness: By creating models that simulate how natural catastrophes affect urban landscapes, generative AI can be used to improve disaster preparedness. These models can be used to find possible weak points and create plans for better catastrophe planning and response. Urban planners may identify structures that are at a high risk of collapsing and determine the importance of retrofitting and strengthening efforts by using Generative AI to create models that replicate the effects of earthquakes on urban buildings.
- Optimising Urban Development: By creating designs that balance different criteria, including population density, transit infrastructure, and environmental effect, generative AI can be utilised to maximise urban development. For instance, AI may produce plans for mixed-use buildings that include residential, commercial, and transportation infrastructure to make cities more livable and sustainable. This can assist cities in accommodating population increase while reducing their negative environmental effects and preserving a good standard of living for their citizens.
Several companies are at the forefront of incorporating generative AI into architecture and urban planning practices. Some notable examples include:
- Digital Blue Foam: Digital Blue Foam is a Singapore-based company specializing in AI-driven solutions for sustainable and efficient urban planning and architectural design. Their flagship product, the Digital Blue Foam web app, streamlines the early stages of the design process by providing architects, designers, and urban planners with a comprehensive and data-driven approach to site analysis and design generation. One of their key features is their generative design capabilities. By incorporating AI-driven algorithms, Digital Blue Foam can generate design options based on user-defined criteria, such as building typologies, density, and energy efficiency. This enables designers to explore multiple design solutions and make informed decisions to optimize their projects. In addition to generative design, they offer features like comprehensive site analysis, which integrates data sources for a thorough understanding of project constraints and opportunities, and a user-friendly parametric design interface for easily customizing designs according to project requirements.
- Delve: Design teams working on urban development projects face various challenges such as the time and cost limitations, complex competing priorities, and unforeseen impacts that may arise from early design decisions. Delve, a generative design software tool launched by Sidewalk Labs, is designed to solve these challenges. Using machine learning, Delve generates a wide range of design possibilities based on the unique criteria of each project, including buildings, open spaces, amenities, streets, and energy infrastructure. This helps design teams to experiment, adjust, and improve designs while responding to community feedback. Delve can help development teams optimize the planning process, exceed their economic goals and improve quality-of-life outcomes for residents and businesses.
- Spacemaker: An offering by Autodesk, Spacemaker is an early urban design and analysis AI programme with a slightly different approach from the AI point of view. It helps urban designers, planners, and real estate developers swiftly mock out and assess various building configurations on a given site. In order to direct the designer towards the best option, it can take into account factors like wind, sun, view quality, and a variety of other criteria. The actual tool is completely web-based. A project is commenced by selecting a site from an interface resembling Google Earth. After the selection is confirmed, your site is generated in a matter of seconds ready for work to begin.
Approaches and Tradeoffs in Using Generative AI in Architecture and Urban Planning
When it comes to using Generative AI in architecture and urban planning, there are various approaches that can be taken, each with its own tradeoffs. In this section, we’ll explore some of these approaches and the tradeoffs associated with them, along with various real-world examples and use cases.
Rule-Based Systems
One approach to using AI Generative design in architecture and urban planning is to use rule-based systems. These systems rely on a set of predefined rules and parameters to generate distinct designs or models. While rule-based systems can be effective in generating simple designs, they may not be able to handle complex design problems that require a more nuanced approach.
For example, in architecture, a rule-based system could be used to generate designs for standard residential buildings. The system would follow predefined rules and parameters, such as the number of bedrooms, washrooms, and living halls/areas, to generate designs that meet these requirements. However, when it comes to more complex designs, such as those for large commercial buildings or public spaces, rule-based systems may not be sufficient. This is because these designs require a more nuanced approach that takes into account factors such as accessibility, sustainability, and user preferences.
Evolutionary Algorithms
Another approach to using Generative AI in architecture and urban planning is to use evolutionary algorithms. These algorithms/techniques mimic the process of natural selection by generating a population of potential designs and selecting the best ones for further refinement. By generating a large number of potential solutions, evolutionary algorithms can help designers explore a wide range of possibilities and identify the most promising ones.
For example, in urban planning, evolutionary algorithms could be used to generate designs for mixed-use developments that balance various factors such as population density, transportation infrastructure, and environmental impact. By generating a population of potential designs and selecting the best ones for further refinement, urban planners can identify designs that optimise these factors and create more livable and sustainable urban environments. However, one tradeoff of using evolutionary algorithms is that they can be computationally expensive and time-consuming. Generating and evaluating a large population of potential designs can require a significant amount of computational power and resources.
Generative Adversarial Networks (GANs)
The third approach to using Generative AI in architecture and urban planning is to use Generative Adversarial Networks (GANs), or a type of deep learning model that can generate new data based on a set of training examples. For example, GANs could be used to generate new designs for buildings or public spaces based on a set of existing designs that are similar in style or function.
However, one vital tradeoff of using GANs is that they can be difficult to control once implemented. Because GANs generate various fresh new designs based on a set of training examples, it can be difficult to ensure that the generated designs meet specific requirements or constraints.
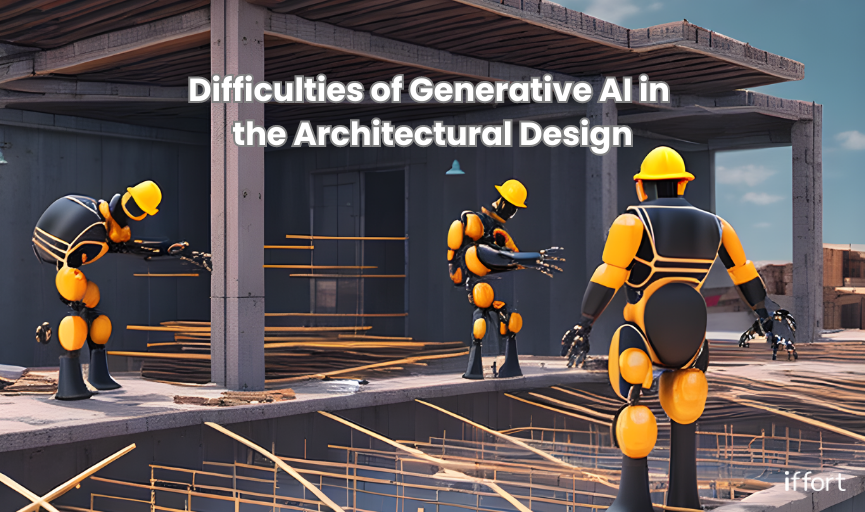
Challenges Associated with Approaches in Generative AI in Architecture and Urban Planning
While there are many benefits to using Generative AI in architecture and urban planning, there are also several challenges that must be considered. In this section, we’ll explore some of these challenges and how they impact different approaches to Generative AI in architecture and urban planning.
- Data Availability: One of the main challenges of using Generative AI in architecture and urban planning is data availability. In order to generate effective designs, the AI model requires a large and diverse dataset to learn from. However, in many cases, such datasets are not readily available, particularly for specific design problems or contexts. For example, in urban planning, there may not be a sufficient dataset of existing mixed-use developments to train an AI model on, thereby limiting the effectiveness of AI-generated designs and making it difficult to create truly innovative solutions.
- Interpretability: Another challenge of using Generative AI in architecture and urban planning is the interpretability of the generated designs. In some cases, it may be difficult to understand how the AI model arrived at a particular design or decision, making it challenging to evaluate or refine the design.
- Ethical Considerations: The use of AI in architecture and urban planning also raises ethical considerations, particularly when it comes to issues of fairness and equity. If an AI model is trained on biased data or makes decisions based on biased criteria, it can perpetuate existing inequalities and contribute to the further marginalisation of certain communities. For example, if an AI model is trained on data that reflects historical patterns of segregation, it may generate designs that perpetuate that segregation, rather than promoting more diverse and inclusive urban environments.
- Computational Requirements: Finally, the use of Generative AI in architecture and urban planning also requires significant computational resources. Generating and evaluating large populations of potential designs can be computationally expensive and time-consuming, particularly for more complex design problems. Training a GAN model, for example, to generate new designs for a complex building or urban environment may require significant computing power and specialised hardware.
As the applications of generative AI in architecture and urban planning continue to grow, partnering with the right technology provider becomes crucial for businesses looking to stay ahead of the curve. At Iffort, we specialise in helping organisations harness the power of generative AI to create groundbreaking solutions tailored to their unique needs.
Our team of experts at Iffort work closely with you to understand your goals and roadblocks and develop custom AI-driven applications that empower your organisation to innovate and excel in the rapidly evolving world of design and urban planning.